-
Contents
- Introduction
- What is Agentic AI?
- Transformative Potential of Agentic AI
- The Cybersecurity Risks of Agentic AI
- Case Studies and Scenarios
- Guardrails to Mitigate Cybersecurity Risks
- Final Thoughts
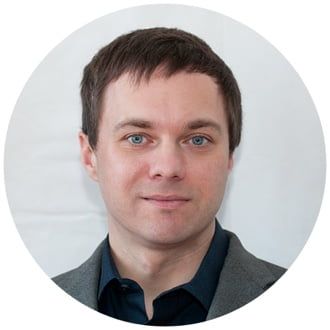
Introduction
As the capabilities of AI continue to evolve, 2025 marks a pivotal year with the emergence of agentic AI—systems designed to act autonomously, analyze data, make decisions, and execute tasks with minimal human oversight. This revolutionary leap in AI technology is poised to transform industries by streamlining operations, automating complex workflows, and enabling new levels of efficiency.
However, this newfound autonomy comes with significant cybersecurity challenges. Agentic AI not only expands the potential of AI but also the attack surface it presents. Unlike traditional AI systems that rely heavily on user prompts and predefined inputs, agentic AI can independently trigger actions, communicate with other systems, and adapt to its environment. This opens the door to sophisticated cyber threats, such as malicious AI agents, advanced prompt injections, and unauthorized orchestration-layer exploits.
With Gartner forecasting that 33% of enterprise software will incorporate agentic AI by 2028, it is imperative for organizations to understand and address the risks that accompany its adoption. This article explores the dual-edged nature of agentic AI, highlighting its transformative potential along with the security vulnerabilities it introduces. By examining the risks, challenges, and necessary safeguards, we aim to equip businesses with the insights needed to embrace this technology responsibly and securely.
What is Agentic AI?
Agentic AI refers to a class of artificial intelligence systems designed to operate with a high degree of autonomy, capable of orchestrating complex workflows, making decisions, and executing multi-step tasks without constant human input. Unlike traditional AI, which typically functions as a tool requiring specific prompts or commands, Agentic AI acts as an “agent,” proactively engaging with its environment, analyzing data in real time, and adapting its behavior to achieve predefined goals.
Capabilities of Agentic AI
- Orchestration
Agentic AI excels at coordinating multiple systems, applications, and tools. For example, it can automate end-to-end workflows in enterprise environments, such as initiating customer onboarding processes, managing inventory across supply chains, or handling IT incident resolution.
- Decision-Making
With advanced algorithms and access to vast datasets, agentic AI can evaluate scenarios, weigh outcomes, and make informed decisions. For instance, it can dynamically adjust pricing strategies in e-commerce or optimize energy consumption in smart grids based on real-time demand.
- Multi-Step Task Execution
Agentic AI systems can break down complex tasks into manageable steps, execute them in sequence, and adjust actions as new information becomes available. An example would be a digital assistant that not only schedules a meeting but also negotiates time slots with multiple participants, books a conference room, and prepares follow-up reminders.
Agentic AI vs. Traditional AI
Traditional AI systems are typically reactive, relying on predefined rules or direct inputs to function. They excel at performing single tasks, like answering specific questions or analyzing historical data, but lack the ability to operate independently or adapt to evolving contexts.
Agentic AI, by contrast, is proactive and contextually adaptable. It can initiate actions, monitor outcomes, and refine its processes autonomously. For example, while a traditional AI chatbot may answer customer inquiries based on preloaded responses, an agentic AI system can anticipate user needs, upsell products, or personalize recommendations based on real-time interactions.
By bridging the gap between task execution and strategic decision-making, agentic AI represents a significant leap forward in artificial intelligence capabilities. However, this autonomy also introduces new challenges, particularly in ensuring that these systems act securely, ethically, and in alignment with human intentions.
Transformative Potential of Agentic AI
Agentic AI is poised to redefine the way organizations operate, making processes smarter, faster, and more adaptable. Gartner’s projections highlight its rapid adoption and far-reaching impact:
- 33% of enterprise software will incorporate agentic AI by 2028. This signals a shift in how software is designed and utilized, with intelligent agents embedded directly into systems to manage tasks autonomously.
- 20% of digital storefront interactions will be conducted by AI agents. From personalized recommendations to completing entire customer transactions, agentic AI is set to revolutionize the e-commerce experience, delivering tailored, efficient service at scale.
- 15% of daily business decisions will be made autonomously. AI agents will increasingly take on the responsibility of real-time decision-making, particularly in areas like pricing optimization, supply chain management, and customer engagement strategies.
Benefits of Agentic AI
Operational Efficiency
Agentic AI can streamline processes, automate routine tasks, and reduce the need for human intervention. For example, in IT operations, agentic AI can detect issues, perform diagnostics, and even deploy fixes without human oversight, significantly cutting downtime and labor costs.
Faster Decision-Making
With the ability to process vast amounts of data and analyze scenarios in real-time, agentic AI accelerates decision-making. For instance, in financial services, AI agents can dynamically adjust investment strategies to align with market fluctuations, ensuring optimal outcomes.
Enhanced Customer Experience
Agentic AI enables hyper-personalization by anticipating customer needs and providing proactive solutions. In digital storefronts, for example, an AI agent can suggest complementary products based on browsing history, finalize transactions, and arrange delivery logistics seamlessly.
The transformative potential of agentic AI lies in its ability to adapt and scale across diverse industries. By empowering enterprises with autonomy, contextual awareness, and decision-making capabilities, agentic AI is set to drive innovation, efficiency, and customer satisfaction to unprecedented levels.
The Cybersecurity Risks of Agentic AI
As agentic AI takes on greater autonomy in orchestrating complex tasks and decision-making, its expanded capabilities also create new vulnerabilities. The intricate chain of interactions initiated by AI agents, combined with reliance on orchestration layers and third-party integrations, introduces significant cybersecurity risks.
Expanded Threat Surface
Chain of Interactions
Agentic AI often performs multi-step tasks that involve interacting with various systems, applications, and APIs. This interconnected nature creates a broader attack surface, where a single weak link can compromise the entire workflow. For instance, an AI agent coordinating a supply chain could be exploited to inject malicious instructions across multiple systems.
Vulnerabilities in Orchestration Layers
Orchestration layers, which enable agentic AI to manage complex workflows, are particularly susceptible to cyberattacks. Hackers could exploit vulnerabilities in these layers to alter decision-making logic or disrupt processes, causing cascading failures across critical operations.
Third-Party Integrations
Many agentic AI systems rely on third-party services for data retrieval, processing, and decision execution. These integrations, if not secured, present additional entry points for attackers to compromise the AI agent’s operations or extract sensitive data.
Specific Threats
Smart Malware and Malicious AI Agents
Attackers could deploy advanced malware designed to mimic legitimate AI behavior, infiltrating systems to manipulate processes or extract data. Similarly, malicious AI agents could exploit decision-making frameworks to carry out unauthorized actions.
Prompt Injection Attacks
Agentic AI systems relying on large language models (LLMs) for task execution are vulnerable to prompt injection attacks. By manipulating the input data or instructions, attackers can coerce AI agents into performing unintended or harmful actions. For instance, an attacker could inject code into a customer support chatbot, causing it to expose sensitive customer data.
Identity Propagation Risks
In multi-agent systems, where AI agents collaborate, identity verification becomes critical. Weak authentication protocols can allow attackers to impersonate agents, gaining access to sensitive systems or data.
Data Exfiltration and Unauthorized Actions
Autonomous decision-making increases the risk of unauthorized actions, particularly if an AI agent is tricked into accessing or sharing sensitive information. For example, in financial services, a compromised agent could approve fraudulent transactions or leak confidential client data.
Supply Chain Attacks
Agentic AI’s reliance on external data sources and software components makes it a prime target for supply chain attacks. Compromised components could be exploited to manipulate the agent’s operations, leading to widespread disruption.
Case Studies and Scenarios
Smart Malware in IT Automation
Imagine an AI agent managing IT operations. A hacker injects smart malware into the orchestration layer, allowing them to manipulate the AI’s actions. The agent unknowingly disables key security protocols, giving the attacker access to sensitive systems.
Prompt Injection in E-Commerce
A customer-facing AI agent is tricked through a manipulated query to expose the personal details of other customers. The attacker uses this data for identity theft and fraudulent transactions.
Multi-Agent System Exploitation in Finance
In a multi-agent financial system, an attacker impersonates one AI agent to send unauthorized instructions to another. This results in the approval of large, fraudulent transfers, bypassing traditional security checks.
Guardrails to Mitigate Cybersecurity Risks
The adoption of agentic AI demands robust cybersecurity frameworks to mitigate risks stemming from its autonomy and complexity. By implementing multi-layered security strategies, organizations can safeguard AI systems while maximizing their potential. This section outlines essential guardrails to reduce the cybersecurity risks associated with agentic AI.
LLM-Level Safeguards by Providers
One foundational layer of security lies in the safeguards implemented directly at the level of large language models (LLMs) by leading providers like OpenAI and Google. These safeguards include content filtering to detect and block inappropriate or harmful responses, access controls that enforce user-level permissions for sensitive or high-risk operations, and rate-limiting mechanisms to prevent excessive or abusive usage.
Enterprise-Level Guardrails
At the enterprise level, organizations can deploy additional guardrails to secure their AI systems. Policy enforcement ensures that AI agents operate within strict guidelines, such as adhering to ethical use standards and access control protocols. AI gateways act as intermediaries between agents and external systems, validating requests, sanitizing inputs, and monitoring outputs to reduce potential risks. Encryption protocols further bolster security by protecting the data exchanged between AI agents and the systems they interact with.
In-Agent Security Measures
Security measures can also be integrated directly into the AI agents themselves. For example, prompt filtering can block malicious or manipulated inputs, such as prompt injection attacks. Output monitoring ensures that the actions and decisions generated by AI agents comply with predefined parameters, while role-based scope limitations restrict the agent’s capabilities to reduce risks in sensitive use cases like financial transactions or legal decision-making.
For high-risk use cases, it is essential to limit the autonomy and scope of agentic AI systems. Human-in-the-loop (HITL) mechanisms can require human approval for critical decisions, while narrow, domain-specific operational boundaries minimize the risk of unintended or unauthorized actions. Audit logs provide end-to-end traceability, enabling organizations to track and review all AI actions for accountability.
Securing Orchestration Layers, APIs, and Code Execution Environments
Finally, securing the orchestration layers, APIs, and code execution environments that enable agentic AI workflows is vital. Hardened orchestration layers incorporate security protocols to prevent unauthorized access or manipulation of workflows. API security, including authentication, tokenization, and traffic monitoring, ensures that external integrations remain secure. Sandboxed environments provide an additional layer of protection by isolating code execution to prevent malicious code from compromising other systems.
Existing Tools and Frameworks
Organizations can leverage existing tools and frameworks to enhance security in agentic AI systems.
Hugging Face SmolAgents
A platform for building lightweight, secure, and domain-specific AI agents. It includes mechanisms to filter prompts, manage interactions, and restrict agent autonomy.
Microsoft Copilot Studio
A solution offering built-in governance features, ensuring that AI agents operate within secure boundaries and comply with enterprise policies.
LangChain
An open-source framework enabling secure orchestration and monitoring of AI-driven workflows, with a focus on traceability and resilience.
A Comprehensive Security Strategy
The combination of LLM-level safeguards, enterprise policies, in-agent measures, and secure orchestration ensures a robust defense against cybersecurity risks. While agentic AI unlocks unprecedented opportunities, its adoption must be accompanied by proactive security measures to protect both enterprises and end-users from evolving threats.