-
Contents
- Harnessing Power
- Mechanics of Change
- Recognition of Images and Presentations
- Driving Innovation
- Overcoming Hurdles
- Road to Success
- Peeking into the Future
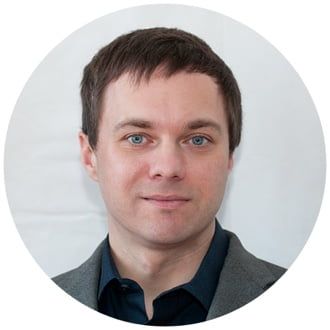
Harnessing Power
As we immerse ourselves further in this digital age, three technologies have begun to substantially stand out: Speech-to-Text Conversion, Generative AI, and Image Recognition. Each of these technologies, when applied independently, bring powerful advantages and opportunities. However, their combined potential is a revolution waiting to happen, enabling capabilities and applications that were once a figment of science fiction.
Speech-to-Text Conversion has been instrumental in fostering a hands-free era of human-computer interaction, allowing us to control devices and dictate messages using our voice. It has numerous applications, from accessibility enhancements to productivity boosts in industries where hands-free operation is beneficial or necessary.
Generative AI signifies a significant stride in artificial intelligence capabilities. No longer confined to rule-based operations, these AI systems can learn from existing data and generate completely new data that mirrors the learned content. This generation can span a multitude of forms – including text, images, and even music – creating a world of possibilities for creative and innovative applications.
Image Recognition, the third pillar of this transformative triad, enables systems to detect and interpret images, thereby understanding the world in a manner similar to humans. It opens a myriad of applications, from tagging photos and scanning barcodes to more advanced uses like facial recognition, object detection, and even disease diagnosis in medical imaging.
But the truly groundbreaking innovation lies at the confluence of these three technologies. When Speech-to-Text Conversion, Generative AI, and Image Recognition are brought together, they create a powerful combination that can transform industries. Imagine a system that can listen to a conversation, convert it to text, understand the context, generate a response, and even visualize data or create relevant images. The potential is vast, and we are just beginning to scratch the surface.
Mechanics of Change
The intersection of Speech-to-Text Conversion and Generative AI is an exciting space teeming with potential. Each technology brings its unique mechanics to the table, creating a formidable combination when applied together.
Speech-to-Text Conversion, or voice recognition technology, forms the foundation of this duo. It is designed to translate spoken words into written text. A complex algorithm processes the audio input, breaking down the speech into phonemes, the smallest units of sound. The system then uses a language model to analyze the context and predict the words that the phonemes are most likely forming. This technology has come a long way, with advancements in machine learning and AI improving its accuracy and enabling it to understand different accents, speech patterns, and even multiple languages.
Generative AI, on the other hand, operates on a different level. It uses machine learning algorithms to understand patterns in the data it is trained on, and then produces new data that mirrors the learned content. In the context of text, it can learn from vast amounts of written material and generate human-like text that matches the style, context, and content of the learned data. Some of the most advanced Generative AI models, such as GPT-4, can draft essays, answer questions, or even create poetry.
The magic happens when these two technologies are combined. Imagine a system that can listen to a spoken conversation, convert it into written text, understand the context, and then generate a meaningful, context-appropriate response. This goes beyond simple command-and-response interactions; we are talking about AI systems that can actively participate in human-like dialogues.
The technologies that underpin Speech-to-Text Conversion and Generative AI are complex and rapidly evolving. Their successful implementation requires deep technical knowledge, as well as a clear understanding of the application’s context and the users’ needs. Despite these challenges, the potential rewards are significant, promising to transform the ways we interact with machines and, ultimately, with each other.
Recognition of Images and Presentations
Adding another dimension to the exciting interplay between Speech-to-Text and Generative AI is the realm of image and presentation text recognition. This innovative application expands the reach of AI capabilities, opening a host of new possibilities and use cases.
This technology, known as optical character recognition (OCR), uses AI to identify and extract text embedded within images or presentations. It can analyze a photo of a document, a slide from a presentation, or even text superimposed on an image, and convert that text into a format that can be edited, searched, and processed further by other AI systems.
The synergy between OCR, Speech-to-Text, and Generative AI is quite potent. For instance, an AI system could analyze the images and slides in a presentation, extract the text, and then use Speech-to-Text conversion to deliver a spoken summary of the presentation’s content. Alternatively, the extracted text could be fed into a Generative AI to create new, related content, such as a written summary, a list of key points, or even a set of potential examination questions based on the material.
Furthermore, the integration of OCR with Speech-to-Text and Generative AI could greatly enhance accessibility, enabling users who have difficulty reading text on screen to access the information in images and presentations through spoken word. It could also facilitate the development of intelligent assistants capable of comprehending and interacting with information in a more holistic way, not just dealing with text or speech in isolation, but integrating understanding from images and visual presentations as well.
In education, business, and many other fields, this combination of technologies could transform how we create, share, and interact with information, making communication more effective, comprehensive, and inclusive. The ability to not only recognize and understand text, whether spoken, written, or embedded within visual media, but also generate new, contextually appropriate content, represents a significant leap forward in AI capabilities.
Driving Innovation
In a diverse array of industries, from healthcare to customer service and education, the fusion of Speech-to-Text Conversion and Generative AI is driving remarkable innovation. These technologies are helping to automate and enhance processes, creating more efficient, intelligent, and user-friendly systems that elevate the user experience and transform operational dynamics.
Consider the healthcare sector, where Speech-to-Text technology can accurately transcribe a doctor’s spoken notes during a patient consultation into written form. Coupling this with Generative AI opens the door to a multitude of innovative applications. The AI could process the transcribed text to generate a comprehensive patient report or extract key points to create a concise summary. It could even generate relevant questions or reminders for the doctor, improving the quality of care and reducing administrative workload.
Customer service is another domain witnessing the transformative power of these technologies. AI chatbots, powered by Speech-to-Text and Generative AI, can understand spoken customer queries, generate appropriate responses, and carry out sophisticated interactions with customers. This not only enhances the customer experience by reducing waiting times and providing 24/7 service but also enables human agents to focus on more complex tasks, improving overall operational efficiency.
The education sector also stands to gain significantly. For instance, an AI-driven system could listen to a lecture, transcribe it, and then generate study notes, summaries, or potential exam questions. It could even create personalized study plans for students based on their individual needs and performance.
Moreover, when paired with image and presentation text recognition, this technology blend can even understand and interact with information within images or slides, making it possible to create intelligent tutoring systems or business intelligence tools with unprecedented capabilities.
Indeed, the marriage of Speech-to-Text Conversion and Generative AI is shaping up as a key driver of innovation, pushing the boundaries of what is possible across sectors and proving that we are only at the beginning of this exciting journey.
Overcoming Hurdles
While the benefits of integrating Speech-to-Text Conversion and Generative AI are vast, the journey to successful implementation is not without its challenges. These obstacles span both technical complexities and broader considerations, such as data privacy and ethical use of AI.
From a technical standpoint, developing systems that effectively combine Speech-to-Text Conversion and Generative AI requires deep expertise in machine learning, natural language processing (NLP), and signal processing. Voice recognition technology, for example, must be capable of accurately transcribing speech across various accents, languages, and environments. Noise reduction, echo cancellation, and speaker identification are all critical factors to consider in ensuring high transcription accuracy.
Generative AI models, on the other hand, have their own set of technical challenges. Training these models to produce high-quality output requires large volumes of high-quality, relevant data. The models must also be carefully designed and tuned to prevent them from merely copying the input data or producing inappropriate or nonsensical output. For example, the use of attention mechanisms in transformer-based models like GPT-4 can help the model focus on the most relevant parts of the input when generating a response.
Another crucial aspect is the integration of OCR technology for image and presentation text recognition. The OCR system must be capable of accurately detecting and transcribing text in a variety of fonts, sizes, and formats, and even in the presence of background noise or distortions.
Beyond the technical aspects, there are also challenges related to data privacy and ethics. When handling sensitive data, such as patient information in healthcare applications or personal details in customer service interactions, it is vital to ensure robust data privacy safeguards are in place. This includes encrypting data, anonymizing personal information, and only using data for its intended purpose.
Moreover, the ethical implications of using AI, especially in applications that interact directly with humans, must also be considered. Issues such as transparency, bias, and accountability need to be addressed to ensure the responsible use of AI.
Despite these challenges, with the right expertise and a carefully planned strategy, it is entirely feasible to develop and implement systems that leverage Speech-to-Text Conversion, Generative AI, and OCR to drive meaningful innovation and progress. It is important to find the right partner to guide you through this journey. If you haven’t found one, our team at Processica is ready to give you a consultation and help you further with implementation.
Road to Success
The journey to successful implementation of a system that leverages Speech-to-Text Conversion, Generative AI, and OCR involves several technical steps and considerations. From understanding the needs of the application to gathering and preprocessing the data, selecting, and tuning the AI model, and continuously testing and refining the system, each step requires careful planning and execution.
The first step is defining clear objectives. What specific task should the system perform? How will it interact with users? What kind of input will it handle, and what should the output look like? The answers to these questions will guide the development process, ensuring the final system meets its intended purpose effectively.
Next, comes the crucial step of gathering and preprocessing the data. For Speech-to-Text Conversion, this could involve collecting audio data in various languages, accents, and environments. For Generative AI, you’ll need large volumes of text data that the model can learn from. In both cases, the data should be diverse, representative, and as clean as possible. Preprocessing might involve removing background noise from audio data or normalizing and tokenizing text data.
Choosing the right AI models is the next significant step. For Speech-to-Text Conversion, deep learning models like recurrent neural networks (RNNs) or transformers have shown remarkable results. In the case of Generative AI, transformer-based models like GPT-4 are typically a viable choice due to their ability to understand the context and generate human-like text. For OCR, convolutional neural networks (CNNs) are often used due to their proficiency in image processing tasks.
Once the models have been chosen, they need to be trained on the preprocessed data. This step could require significant computational resources, especially for larger models and datasets. After training, the models should be validated and tested on unseen data to evaluate their performance.
Finally, once the system has been developed and tested, it should be continuously monitored and updated, as necessary. AI models can drift over time as they encounter new types of data, so it’s important to regularly retrain them to ensure they continue to perform well. It’s also crucial to update the system as new versions of the AI models become available.
Implementing a system that harnesses Speech-to-Text Conversion, Generative AI, and OCR is a complex task that requires a strong understanding of AI and machine learning principles, as well as the specific requirements of the application. However, the rewards of navigating this road to success can be substantial, offering transformative potential across a wide range of sectors and applications.
Peeking into the Future
As we venture into the future of Speech-to-Text Conversion and Generative AI, we stand at the precipice of uncharted territory, ripe with potential and marked by significant technological advancement.
The future of these technologies is set to revolve around increased accuracy and sophistication. For Speech-to-Text Conversion, we can expect advancements in machine learning algorithms that lead to improved accuracy in transcribing speech, even in challenging environments or among speakers with heavy accents. We might also see improvements in the technology’s ability to understand context and nuance in spoken language, even detecting and interpreting emotional cues.
Generative AI is set to become more nuanced and contextually aware. Advancements in AI architectures, like transformer-based models, will likely enable more human-like text generation. Generative AI will become better at understanding context, maintaining thematic consistency, and even being creative. It might also develop an ability to generate content in other formats like images or music, based on the text it has learned from, opening up even more potential applications.
With image and presentation text recognition, we can anticipate improvements in the accuracy of OCR systems, their ability to understand complex layouts, and recognize text in multiple languages or scripts. As machine learning techniques become more advanced, we might see systems that can not only recognize and extract text from images or presentations but also understand the underlying context or narrative.
Furthermore, we can expect to see advancements in the ways these technologies interact. The integration of Speech-to-Text Conversion, Generative AI, and OCR could lead to the development of more sophisticated and versatile AI systems. For instance, we might see AI tutors that can deliver personalized education, understanding and responding to spoken questions, providing explanations based on a range of sources, and even generating tests based on the student’s learning progress.
However, along with these advancements come challenges. The technical complexities of these technologies will continue to grow, requiring more sophisticated models, larger datasets, and more computational power. Ethical and privacy concerns will also need to be addressed, particularly as these AI systems become more integrated into our daily lives.
Despite these challenges, the future potential of combining Speech-to-Text Conversion with Generative AI, bolstered by the power of OCR, promises to make a significant impact across industries. From healthcare and education to customer service and beyond, the possibilities are expansive, with the promise of innovation and transformation at every turn.